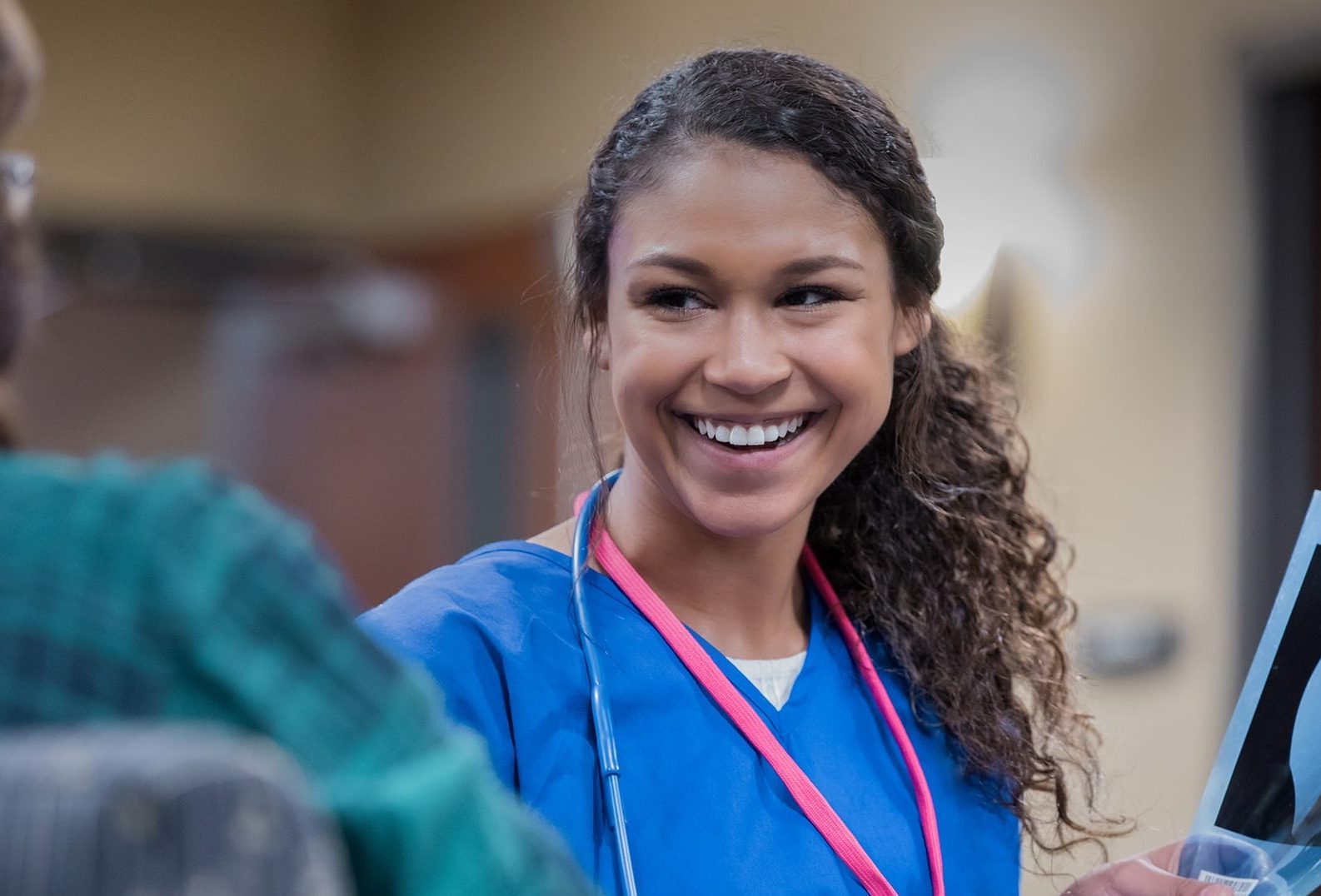
NHS Code of conduct for data-driven health and care technology
The initial code of conduct for data-driven health and care technology, released on the 5th September 2018, contains ten principles that set out what the UK Department of Health and Social Care expect from suppliers of data-driven technologies.
This document is Kheiron’s initial response to those principles, to assure our NHS partners and the public that we offer safe, effective and secure technology aimed at improving NHS services. Please note that this document represents only a preliminary draft of our responses to the guidance from the Department of Health and Social Care. We are committed to abiding by this code of conduct and developing our response over the coming months as we pride ourselves on robust clinical validation and being vigilant in our approach. We welcome feedback on this initial response at [email protected].
Resources
Initial code of conduct for data-driven health and care technology – 5th Sept 2018.
10 principles
1 Define the user
The UK is facing a workforce crisis in radiology. There are simply too few radiologists to keep up with demand. This is especially acute in breast cancer screening, where 25% of units have two or fewer breast radiologists. Further, between now and 2022, for every two breast radiologists that join the NHS, three are predicted to leave.
There are 80 Breast Screening Units in England. The total number of women invited in 2016/17 in England for Breast Screening rose by 3.7% to 2.96 million. Of those, 2.2 million accepted the invitation and were screened, and 18,402 cancers were detected. Currently every mammogram is ‘double-read’ by two independent radiologists, however due to stretched staffing levels, many screening centres struggle to fulfil double reading requirements in a timely manner. Additionally, there is wide variation in recall rates between different centres.
The users in our case are therefore breast screening programmes and breast radiologists seeking to support their work in mammography screening for the benefit of patients.
2 Define the value proposition
Kheiron’s deep learning tool is intended to analyse mammograms just like an independent reader within existing screening programmes and services, acting as a second or third reader along side radiologists, overseen by standard arbitration processes, thus potentially increasing throughput and screening performance. It is therefore used in conjunction with an expert-level radiologist within existing PACS and radiology reporting systems. Initial studies have demonstrated a sensitivity and specificity for case-wise screening recall decisions that compare favourably to established screening performance benchmarks with 100% repeatability.
While there is no point of reference for the deployment model of a comparable technology in the UK, Kheiron intend to work with our UK partner sites, radiologists and patients, together with health economists to refine our approach for optimal feasibility and effectiveness. Our software has the potential to slot into the radiology workflow at a number of different points e.g. as a second or third reader, and as a prioritisation tool, or quality control tool.
3 Be fair, transparent and accountable about what data you are using
Kheiron is registered with the Information Commissioner’s Office (ICO). Prior to working on any clinical data, we work closely with our clinical partners to ensure that privacy-by-design comes first and foremost by ensuring the relevant impact assessments, research/ethical approvals and local information governance policies and procedures have been adhered to and completed where necessary. We have developed the required data flow mappings, clinical study protocols, data management plans and System Level Security Policy (SLSP) that is designed to comply with ISO 27001 certification, and ask our clinical partners to complete a Data Privacy Impact Assessment (DPIA) for their organisation.
Data-sharing agreements are founded on a legal basis for data processing. Depending on the context and the type of partner (including if they are a public or private body), partners may consider utilising specific articles from GDPR as their lawful basis for sharing de-identified data with us.
4 Use data that is proportionate to the identified user need (data minimisation principle of GDPR)
We only use data that is relevant to our research, and we never use personally identifiable data. We actively restrict our own access to information and data for the purposes of privacy and data security but also for the purpose of keeping with good machine learning principles and clinical practice.
5 Make use of open standards
Our deep learning tool has been developed to use the current edition of the DICOM image standard, the international standard to transmit, store, retrieve, print, process, and display medical imaging. Using the DICOM standard allows us to integrate our product with existing Picture Archive and Communication Systems (PACS) and clinical-grade image viewers. We are also guided by DICOM supplement 142: Clinical Trial De-identification Profiles in the preparation of our de-identification tools.
Kheiron have developed several proprietary processes internally to ensure data quality and security.
Kheiron welcome any further guidance from NHS Digital on which standards technology SMEs creating data-driven technology should be utilising.
6 Be transparent to the limitations of the data used and algorithms deployed
Our approach is to conduct several phases of clinical validation on high-quality well-curated real-world data as close to our intended screening population cohort as possible. Our phase I study was a retrospective validation study performed on a statistically powered cohort of real-world mammography cases. This involved de-identified data from patients who had attended European sites for mammography screening, with clear inclusion and exclusion criteria applied.
The data used in this initial study represents real-world screening cases, but is limited to images that were acquired from mammography machines of one hardware vendor at European screening centres. Results are pending publication in a peer-reviewed journal. The limitations of our system are clearly defined in full in our product labelling (for example, it should not be used to analyse mammography images from males). Ongoing future work is aimed at eliminating relevant limitations.
7 Make security integral to design
We have an internal System Level Security Policy (SLSP) that is designed to comply with ISO 27001 certification. We also take a risk-based approach to ensure appropriate safeguards and level of security are in place to keep systems safe.
In summary, we employ strict encryptions, and engage technical and, where possible, physical layers of security to prevent unauthorised users both within our team and externally from having access to data. We do not see or handle identifiable patient data at any point. We have strict access control tools and infrastructure in place. We have logging and monitoring tools that are used to flag any suspicious activity. We have internal procedures to deal with security incidents and procedures to manage changes to our system.
8 Define the commercial strategy
Regarding commercial strategy with the NHS, our analyses suggest that the positive effects of deep learning assisted screening programmes on downstream patient pathways could save the NHS significant costs. We intend to work with our UK clinical research partners to fine tune our business model for the NHS, by undertaking independent health economics analyses. Our research and development work with our clinical partners is grant supported to ensure that our clinical partners have the resources and funding needed to support the required effort. For example, we are taking part in an NHS England Wave 2 Test Bed project with the East Midlands Radiology Consortium (EMRAD).
9 Show evidence of effectiveness for intended use
Our initial study of our deep learning software enabled application for, and subsequent granting of a CE mark as a medical device.
Now, we are conducting a second phase of clinical studies on a widened cohort of mammography screening data from across the NHS and Europe, so as to ensure the robustness of our software’s performance in diverse conditions. Pending these results, our CE mark will be updated accordingly.
Due to the nature of deep learning and its potential to improve over time when given more data, it is important that our partners are kept up-to-date regarding our software’s performance. Our clinical research partners receive documentation and information regarding the accuracy and evidence generated to date regarding our software, and are actively involved in it’s assessment and studies.
10 Show what type of algorithm you are building, the evidence base for choosing that algorithm, how you plan to monitor its performance on an ongoing basis and how you are validating performance of the algorithm.
Our algorithms are built on a complex interlink of Convolutional Neural Networks (CNNs), which pre-process, analyse and interpret standard DICOM images (mammograms) on a case-wise basis. CNNs are the backbone of many of the recent advances in image analysis.
Validation is ongoing in phases of clinical studies and trials. We have completed the NICE Medtech Early Technical Assessment (META) tool and have received expert feedback from NICE, which we are using to inform our plans for further evidence generation. Our UK studies are also registered with and supported by the NIHR Clinical Research Network, and we have sought support from Public Health England. Furthermore, we have strict internal benchmarking systems and data quality control processes in place. Post market surveillance and audits will be conducted after deployment in keeping with ISO 13485.
Summary statement
We believe that being vigilant in our approach to developing our deep learning tools, and making sure our algorithms are appropriately and rigorously clinically validated, is in Kheiron’s DNA. We are committed to abiding by the principles set out by the Department of Health and Social Care and would welcome any feedback on this initial response. We also aim to be open and transparent in the wider academic community regarding our software. For instance, we aim to present our results at relevant academic conferences, and we intend to publish all study and trial results in peer-reviewed journals.